# Streaming Non-Monotone Submodular Maximization: Personalized Video Summarization on the Fly | Proceedings of the AAAI Conference on Artificial Intelligence #paper
1. paper-info
1.1 Metadata
- Author:: [[Baharan Mirzasoleiman]]
- 作者机构:: 加州大学
- Keywords:: #Submodular , #VideoSummarization
- Journal:: #AAAI
- Date:: 2019
- 状态:: #Doing
1.2 摘要
The need for real time analysis of rapidly producing data streams (e.g., video and image streams) motivated the design of streaming algorithms that can efficiently extract and summarize useful information from massive data “on the fly”. Such problems can often be reduced to maximizing a submodular set function subject to various constraints. While efficient streaming methods have been recently developed for monotone submodular maximization, in a wide range of applications, such as video summarization, the underlying utility function is non-monotone, and there are often various constraints imposed on the optimization problem to consider privacy or personalization. We develop the first efficient single pass streaming algorithm, STREAMING LOCAL SEARCH, that for any streaming monotone submodular maximization algorithm with approximation guarantee α under a collection of independence systems I, provides a constant 1/(1+2/√α +1/α +2d(1 + √α)) approximation guarantee for maximizing a non-monotone submodular function under the intersection of I and d knapsack constraints. Our experiments show that for video summarization, our method runs more than 1700 times faster than previous work, while maintaining practically the same performance.
efficient streaming function
,monotone submodular maximization
,single pass streaming algorithm
2. Introduction
data summarization
:从大型数据集中有效提取能够表征大数据集且课管理大小的子集的任务。
Submodular maximization
:解决data summarization
的手段。
data summarization can be naturally reduced to a constrained submodular optimization problem
- 任务:
In particular, we consider video summarization in a streaming setting, where video frames are produced at a fast pace, and we want to keep an updated summary of the video so far, with little or no memory overhead.
对视频流进行概括,保证效率的同时不牺牲内存。
- 问题:
- 没有高效的流式非单调次模最大化方法,在很多约束下。
- 解决办法:
Streaming Local Search algorithm
:只需要对数据进行一次传递。本研究提出了一个通用框架,在该框架下,可以使用任意的streaming monotone submodular maximization algorithm
3. Streaming algorithm for constrained submodular maximization
constrained:
- independence systems
- d knapsack constraints
3.1 Streaming Local Search for a collection of independence systems
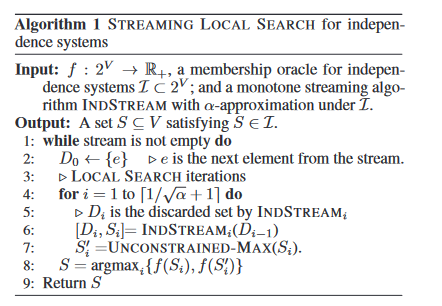
3.2 STREAMING LOCAL SEARCH for independence systems and multiple knapsack constraintsdence
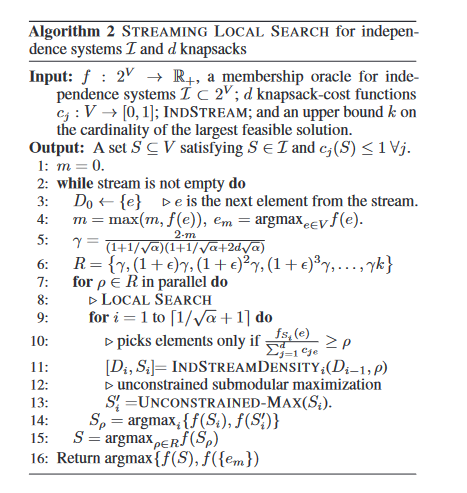
4. Experiments
- Dataset
- Open Video Project(OVP)
- YouTuBe datasets
5. 总结
没有了解论文具体细节,只是简单了解submodular function
的应用。该文章属于有约束的非单调次模函数最大化问题,且是流式。算法过程如Figure 2
所示。
原文地址:http://www.cnblogs.com/guixu/p/16819200.html